SynapseNet: Deep Learning for Automatic Synapse Reconstruction
Posted on 24 February 2025
Electron microscopy provides unmatched detail for studying synaptic morphology, but manual segmentation is a bottleneck. SynapseNet is a deep-learning tool that automates not only synapse reconstruction but also efficient, scalable analysis. It is trained on a large annotated room temperature EM dataset and can segment structures such as synaptic vesicles (SV), mitochondria, active zones (AZ), and compartments. With domain adaptation functionality, it also reliably segments SVs across a wide range of imaging techniques and data domains.
For more details on SynapseNet check out the software at https://github.com/computational-cell-analytics/synapse-net and the preprint at https://doi.org/10.1101/2024.12.02.626387.
Contact email: constantin.pape@informatik.uni-goettingen.de
Resource Created By: Sarah Muth, Frederieke Moschref, Luca Freckmann, Sophia Mutschall, Ines Hojas-Garcia-Plaza, Julius N. Bahr, Arsen Petrovic, Thanh Thao Do, Valentin Schwarze, Anwai Archit, Kirsten Weyand, Susann Michanski, Lydia Maus, Cordelia Imig, Nils Brose, Carolin Wichmann, Ruben Fernandez-Busnadiego, Tobias Moser, Silvio O. Rizzoli, Benjamin H. Cooper, Constantin Pape
Type of tool: Image analysis software
Is this tool open source?: Yes
You may also be interested in
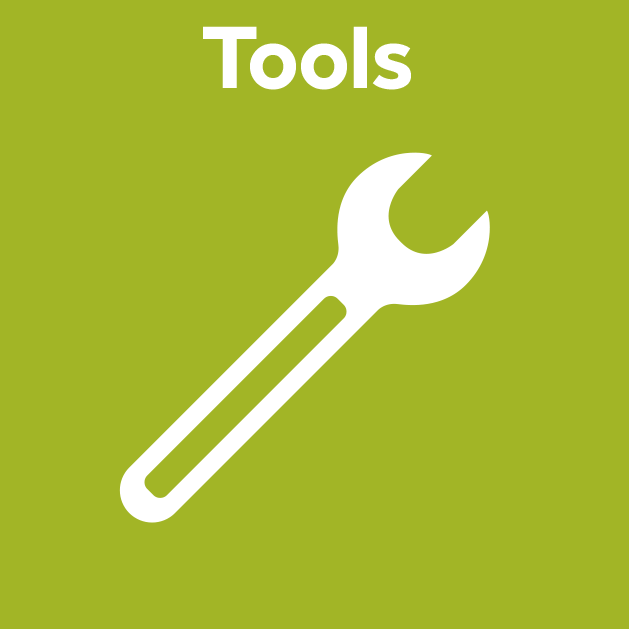
The Open Microscopy Environment
Tools to support data management
University of Dundee & Open Microscopy Environment
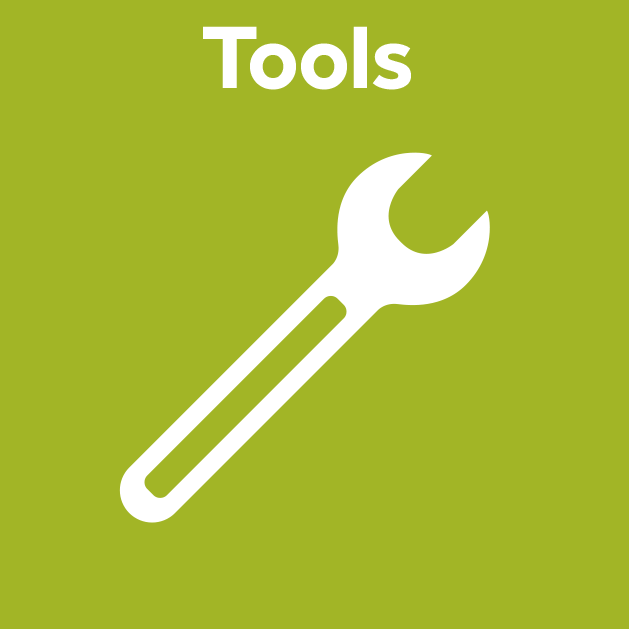
SynapseNet: Deep Learning for Automatic Synapse Reconstruction
automatic synapse reconstruction and analysis in EM
Sarah Muth, Frederieke Moschref, Luca Freckmann, Sophia Mutschall, Ines Hojas-Garcia-Plaza, Julius N. Bahr, Arsen Petrovic, Thanh Thao Do, Valentin Schwarze, Anwai Archit, Kirsten Weyand, Susann Michanski, Lydia Maus, Cordelia Imig, Nils Brose, Carolin Wichmann, Ruben Fernandez-Busnadiego, Tobias Moser, Silvio O. Rizzoli, Benjamin H. Cooper, Constantin Pape
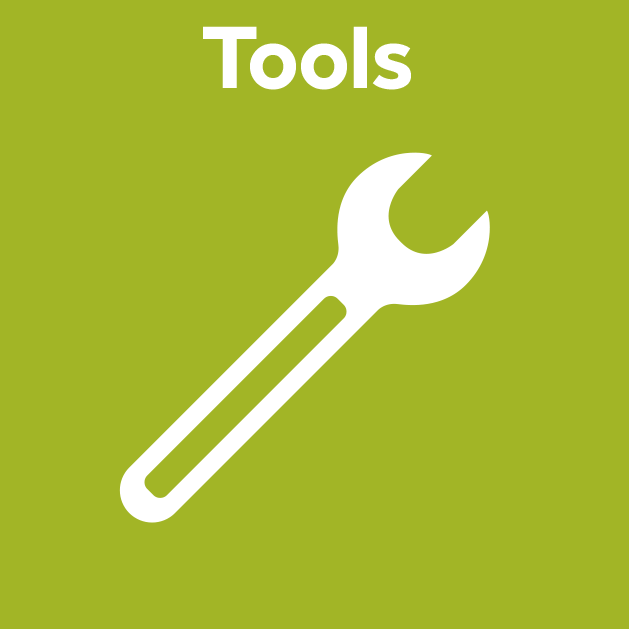
Microscopy Image Browser
MATLAB-based tool for advanced image processing
Ilya Belevich, Merja Joensuu, Darshan Kumar, Helena Vihinen and Eija Jokitalo