Enhancing Global Access: interview with CZI grantee Thierry Pecot
Posted by Mariana De Niz, on 24 January 2024
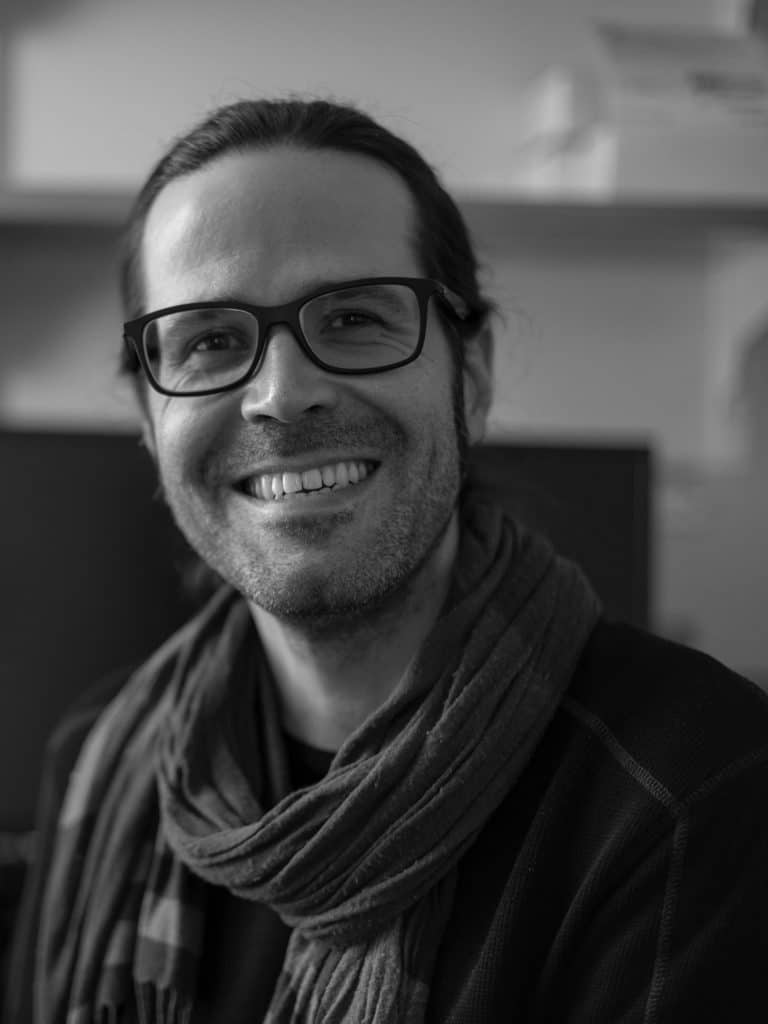
BRINGING ARTIFICIAL INTELLIGENCE TO BIOLOGISTS
Thierry Pécot is an engineer and applied mathematician who develops deep learning frameworks for a variety of applications. As his project for the Cycle 1 imaging scientist call, Thierry Pécot proposed enabling biologists to apply deep learning tools to their own images. He interacts with biologists to identify the needs required to develop deep learning suits for large scale segmentation. He also developed courses and tutorials to bridge the gap in expertise in biomedical image analysis. He just created the new shared facility FAIIA, dedicated to artificial intelligence and image analysis applied to microscopy..
What was the inspiration for your project? How did your idea for the CZI project arise?
I started this project when I was in Charleston in South Carolina. I was working half of my time in a molecular biology lab, and half of the time in a shared facility, developing new tools for them to use. Then there was the call for the CZI imaging scientist grant and I used this to grow more into the shared facility service.
What do you think is the value of core facilities?
Core facilities enable us to have expertise at a large scale for lots of scientists. For example, in my current institute in France, I am the only person with expertise on campus, so without a shared facility, there would be no one to provide this service. Core facilities help expand access to expertise and resources.
When did you first hear about the CZI program and how did you decide to apply to it?
I was very lucky, one of my colleagues sent me an email telling me about the call and suggesting I should apply. My current grant ends next June and I am now negotiating with the university a permanent position.
You are in a discipline that bridges gaps in knowledge, helping biologists do complex pipelines for image analysis. You have worked on the ICY project and other tools. What do you think is the future of bridging expertise so that any biologist in the future can do image analysis? Do you see this as part of ensuring global access?
Indeed, that is what I’m trying to do here. I offer 3 different types of training: one covering the basics of Fiji (7 sessions of 2 hours each), one on QuPath (2 days) and one on training deep learning models for image classification and segmentation (3 days). The last one is the most evolved, since I have been doing it for several years. I have always had a lot of attendees. A large number of people who attend are there because they want to learn and understand how AI works, from basic concepts to advanced applications. Some go on to use the methods on their own projects. I feel that bridging the gap is certainly not easy, but it is doable. I feel things are changing, though, in that PhD students and other young scientists are more willing to learn about these topics – they grew up in a digital environment, they feel less afraid about it, and education now integrates this important skillset earlier on.
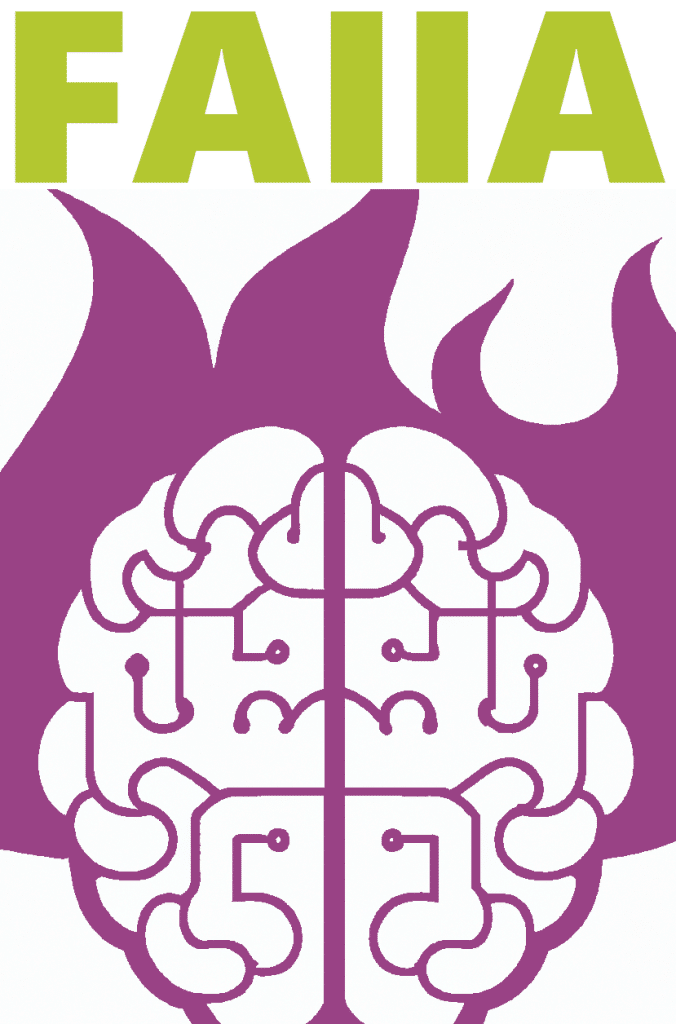
What challenges have you faced during your CZI project?
I think the concept people have about image analysis is sometimes challenging. Sometimes people are appealed by the technology side, they acquire images, and then ask me if I can analyze the data. But if I don’t know their research question, their hypothesis, what they want to quantify, or how they acquired the images, it’s difficult for me to help. I think it is important to educate people on how to formulate hypothesis and how to integrate imaging in the scientific method of their experimental design. You need to know what to do with the data, because with the same data you can say many different things.
What are the lessons you have learned from your project, and what are your top successes?
The biggest success is training people. I can share a great example of this. When I arrived here, there was a user, a PhD student who wanted to quantify various things. She was using code that an intern in the lab who left before she arrived, had started to do in Python. The PhD student didn’t know how to code, and she told me she wanted to learn Python, so I recorded everything and we went through it together. Six months later I saw her again and she told me the pipeline was successful but she wanted to see what else could be integrated and/or improved. She wanted to see whether she could automatically identify the cells of interest without the fluorescent markers originally used to identify the cells. She went over tutorials on the internet to try and learn how to do machine learning, and she applied this approach to identify cells of interest. I think it was very cool that we started working together and then she independently gained expertise on more sophisticated tools and methods.
What is the unique value and impact of the CZI program? Is there something that makes it unique compared to other grants or other types of funding?
The CZI grant gives you a lot of time and money to spend on things that you think are important. For example, the trainings I give here, I would never have had the time to develop them without the grant. You don’t have to worry about your salary to develop your ideas. You can focus on things that really matter to you. In my proposal there was a focus on developing training programs on AI techniques, image analysis techniques and developing new methods. Without the grant, I might have had time to develop new methods because I don’t have a choice, since I need to publish. But clearly I would not have been able to focus on developing new training courses.
Beyond funding, how has the grant helped you reach your aims and objectives for the project?
It has been very helpful to meet with other grantees. We have a lot of funding to travel, which allows us to attend the CZI conferences and meetings. This allows us to meet grantees in the same position who are focusing on microscopy and image analysis. The goal of these meetings is to find that the problems are in the different disciplines, and identify bottlenecks to advance research, especially when you are working in a facility. These are questions that we otherwise never had the opportunity to ask ourselves, let alone address. Being in an environment with people who have a similar vision and are willing to exchange ideas has been really helpful. CZI has also funded networks like Bioimaging North America (BINA) and Latin America Bioimaging (LABI), which also foster collaborations and relationships. I was invited to the BINA Training and Education working group, and so I also get the chance to engage there.
What future do you envisage for your project? Where do you want it to be in 10 years?
The idea is to create a strong shared facility for image analysis and advanced microscopy. We are lucky because in France, France Bioimaging has a network of image analysts. We are in contact with each other and this allows us to communicate and share experience and expertise. The goal is to have something that is helpful on campus and beyond, for people that don’t currently have access to image analysis expertise or tools. The more we give trainings and the more people we meet, the more sophisticated things we will be able to do. Eventually we could reach the level of what people are doing in the field of Computer Vision.
Check out our introductory post, with links to the other interviews here