Part V The future: The hope of smart microscopes and phantoms
Posted by Elisabeth Kugler, on 24 April 2021
Elisabeth Kugler 1 and Emmanuel G. Reynaud 2
Contact: kugler.elisabeth@gmail.com; emmanuel.reynaud@ucd.ie
We are reaching the end of our LSFM journey. Now it is time to look into the future, riding the plane of light! So, what is next for the LSFM?
1. Four-to-two, then four, then one.
The story of SPIM, and LSFM per se, started in 4Pi microscopy with four objectives, but a twist in the tale reduced it to two and SPIM was born and published in 2004 [1]. Since then, more than 140 acronyms have been coined for all sorts of variants. Complexity and capability lead to systems of up to four objectives that were published, patented, and commercialized.
However this year, Nature Methods published a delightful column on how single objective LSFM may be the future of the field [2]. It is true that their versatility does fit the flat and horizontal mounting of many established sample preparation methods involving slides, dishes, or plates. Improved sample mounting ergonomics promises to facilitate the adoption of LSFM and the jump to the magic of 3D imaging. It also brings speed (SCAPE) and amazing optics (MrSnouty).
2. Smart microscopy
LSFM does generate a lot of data and as we discussed in previous posts, often this manifests itself at the start of the process, namely during image acquisition. Acquisition in LSFM is also plagued by many issues, such as refraction or scattering, caused by the propagation of the light sheet through the sample. Thus, it is worth investing in a techniques able of improving image quality during and post-acquisition [3]. Nowadays so-called smart microscopes can optimize imaging steps and reduce data load. This is achieved by tailoring the imaging volume to the sample as well as by ensuring optimal illumination, view numbers, angles, and optimal acquisition strategy. It is possible to think about a LSFM smart microscope to being able to optimize data acquisition (e.g. adaptive optics) as well as data pre-processing (e.g. cropping or down-sampling) [4]. A combination of these features might be a winning strategy as LSFM microscopy is not a single step but an integrated element of a microscopy protocol.
3. Variable deconvolution
Well, we cannot ignore the artificial intelligence (AI) in the room. As LSFM uses a light sheet shining through the sample and an orthogonally positioned detection path, it is affected by signal degradation along the two main optical paths: illumination and detection. These optical effects cause artefacts and degrade the point-spread-function (PSF). The usual suspect to correct these issues is deconvolution, but as PSF variation across the volume is usually non-linear, the use of “variable” deconvolution may be a useful tool and AI may be the way forward.
AI could also be useful to improve image quality by processing other artefacts, such as background. This can be particularly important when using more exotic illumination approaches like Bessel Beams. Many approaches have been published and software and microscope manufacturers have implemented some, but it still requires work and optimization as there are vast amounts of LSFM implementation, yet comparative samples and datasets are still missing to allow for direct comparisons.
4. Image feature extraction and analysis
To further reduce data load and establish new ways of analysis, one can also move away from visual images to other mathematical representations, such as point clouds or graph (networks). Abstracting image data in this way reduces data load and unlocks new levels of analysis (beautifully demonstrated in Hartmann et al., 2020 [5] and Machado et al., 2019 [6]). Moving away from voxel-based 3D/4D/XD data to numerical features allows for a new understanding to be extracted using computational tools from statistics and machine learning, which in turn enables advanced image processing such as feature-based registration (see Neubias Academy at Home). However, more work is needed to make feature extraction, point clouds, and graph representation more accessible to biologists.
5. (Optical) Phantoms and benchmark datasets
Our last suggestion (at least within this series) are optical phantoms that mimic tissue/organs/organisms and benchmark datasets [7]. While certain fields, such as medicine, apply phantoms as a standard to test image acquisition and data analysis workflows, this is less widely applied in biomedical sciences. Often data are accepted as “ground truth” without phantoms, comparisons of methods, manual grading, or manual segmentation. This mistake is particularly evident when looking at the point-spread-functions obtained by imaging fluorescent beads that could be used as phantoms.
Unfortunately, it does not end here. Indeed, we need benchmark datasets and further studies that will allow the comparison of image analysis workflows across labs and computing languages. Efforts to bridge these gaps are exemplified by projects such as clEsperanto , which bridges computing languages.
Together, we have taken you on a journey covering various aspects of LSFM, starting from sample preparation, to artefacts, data handling, and the future. We hope you enjoyed this endeavour as much as we did!
Again, huge thanks to all colleagues who provided feedback and the fantastic FocalPlane team, particularly Christos Kyprianou and Esperanza Agullo-Pascual.
Our final: may the LISH be with you,
Elisabeth and Emmanuel
P.S.: If you want to share your views, do not hesitate to get in touch.
Acknowledgements
We are incredibly grateful to Jonas Hartmann and Loïc Royer for their invaluable feedback.
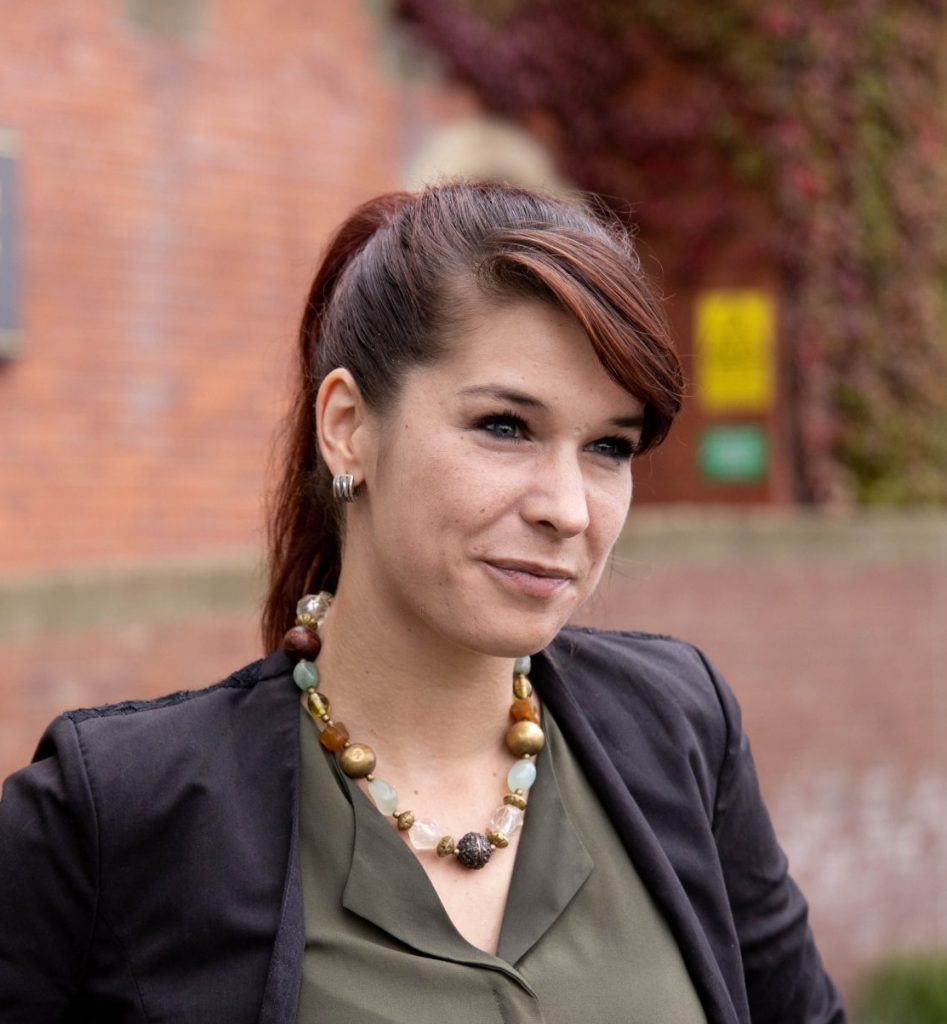
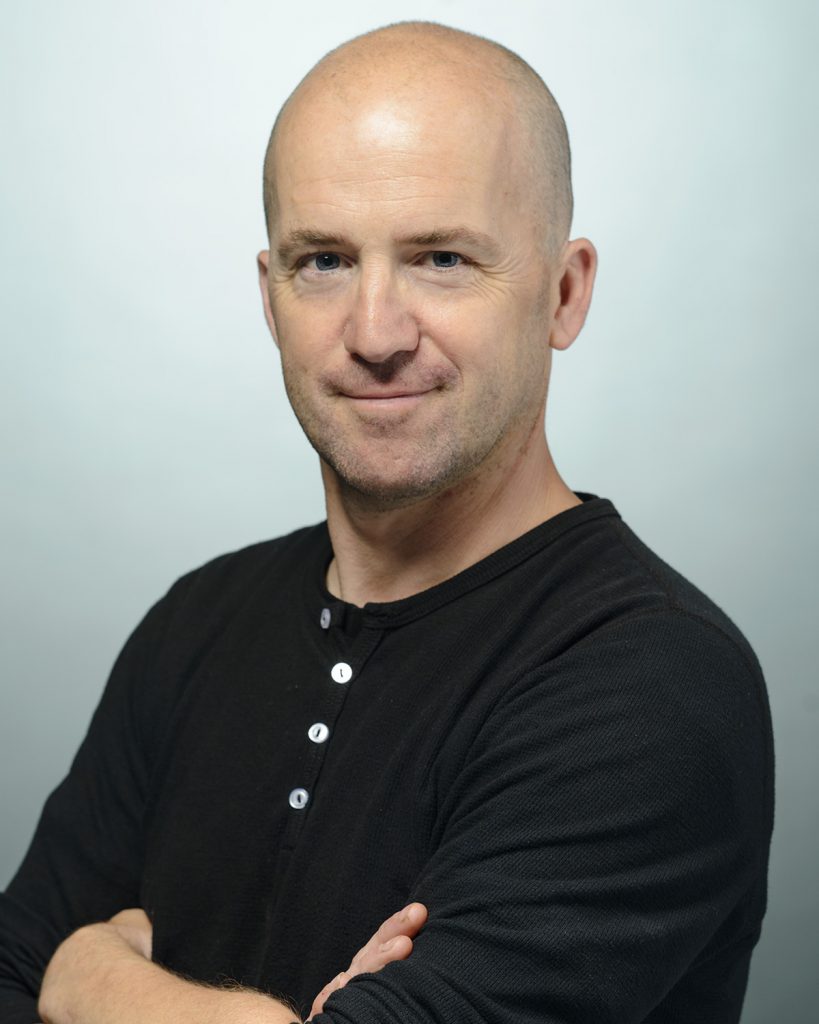
Associations / Institutes
(1) Institute of Ophthalmology, Faculty of Brain Sciences, University College London, 11-43 Bath Street, LondonEC1V 9EL. (2) School of Biomolecular and Biomedical Science, University College Dublin, Belfield, Dublin 4. Ireland.